Catherine D’Ignazio and I just presented a paper titled “Approaches to Big Data Literacy” at the 2015 Bloomberg Data for Good Exchange 2015. This is a write-up of the talk we gave to summarize the paper.
When we talk about data science for good, collaborating with organizations that work for the social good, we are immediately entered into a conversation about empowerment. How can data science help these organizations empower their constituencies and create change in the world? Catherine and I are educators, and strongly believe learning is about empowerment, so this area naturally appeals to us! That’s why we wrote this paper for the Bloomberg Data for Good Exchange.
Data Literacy
We’ve been thinking and working a lot on data literacy, and how to help folks build their capacity to work with information to create social change. We define “data literacy” as the ability to read, work with, analyze and argue with data. So how do we help build data literacy in creative and fun ways? One example is the activity we do around text analysis. We introduce folks to a simple word-couting website and give them lyrics of popular musicians to analyze. Over the course of half and hour folks poke at the data, looking for stories comparing word usage between artists. Then they sketch a visual to share a story.
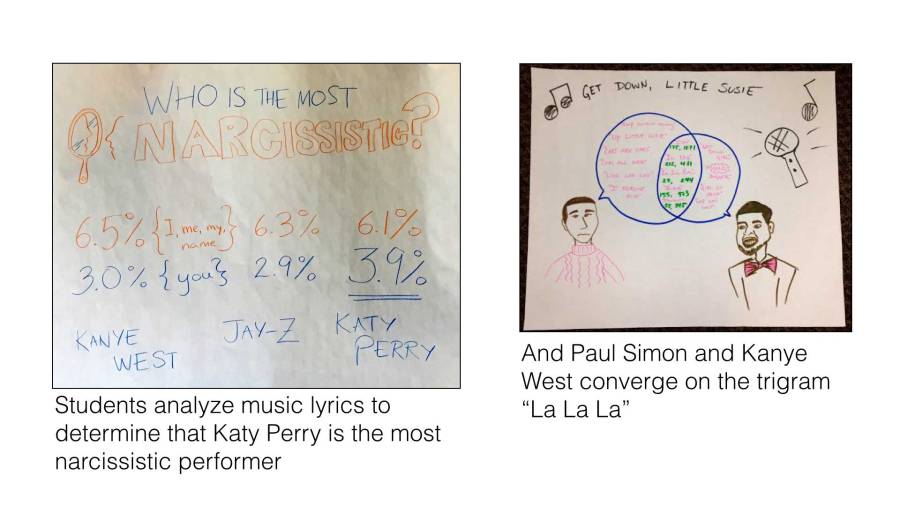
Another example are my Data Murals – where we help a community group find a story in their data, collaboratively design a visual to tell that story, and paint it as a community mural.
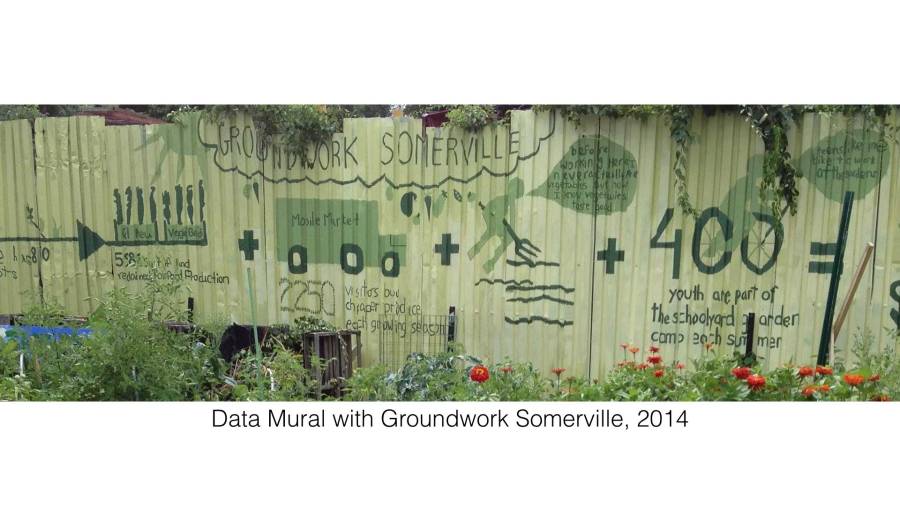
This stuff is fun, and makes learning to work with data accessible. We focus on working with technical and non-technical audiences. The technical folks have a lot to learn about how to use data to effect change, while the non-technical folks want to build their skills to use data in support of their mission.
Empowerment
However this work has been focused on small data sets… when we think about “big data literacy” we see some gaps in our definition and our work. Here are four problems related to empowerment that we see in big data, related to our definition of data literacy:
- lack of transparency: you can’t read the data if you don’t even know it exists
- extractive collection: you can’t work with data if it isn’t available
- technological complexity: you can’t analyze data unless you can overcome the technical challenges of big data
- control of impact: you can’t argue for change with data unless you can effect that change
With these problems in mind, we decided we needed an expanded definition of “big data literacy”. This includes:
- identifying when and where data is being collected
- understanding the algorithmic manipulations
- weighing the real and potential ethical impacts
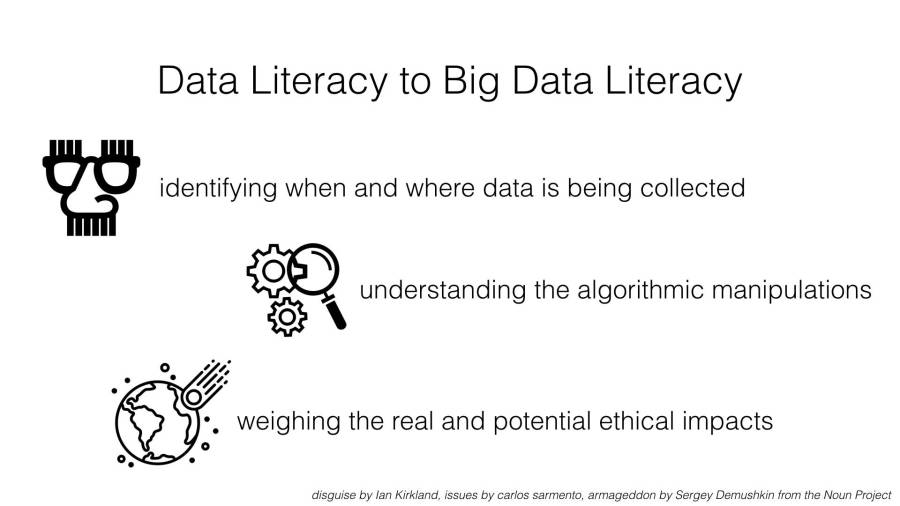
So how do we work on building this type of big data literacy? First off we look to Freire for inspiration. We could go on for hours about his approach to building literacy in Brazil, but want to focus on his “Population Education”. That approach was about using literacy to do education and emancipation. This second piece matters when you are doing data for good; it isn’t just about acquiring technical skills!
Ideas
We want to work with you on how to address this empowerment problem, and have a few ideas of our own that we want to try out. The paper has seven of these sketched out, but here are three examples.
Idea #1: Participatory Algorithmic Simulations
We want to create examples of participatory simulations for how algorithms function. Imagine a linear search being demonstrated by lining people up and going from left to right searching for someone named “Anita”. This would build on the rich tradition of moving your body to mimic and understand how a system functions (called “body syntonicity“). Participatory algorithmic simulations would focus on understanding algorithmic manipulations.
Ideas #2: Data Journals
Data can bee seen as the traces of the interactions between you and the world around you. With this definition in mind, in our classes we ask students to keep a journal of every piece of data they create during a 24 hour period (see some examples). This activity targets identifying when and where data is being collected. We facilitate a discussion about these journals, asking students which ones creep them out the most, which leads to a great chance to weigh the real and potential ethical implications.
Ideas #3: Reverse Engineering Algorithms:
We’ve seen a bunch of great work recently on reverse engineering algorithms, trying to understand why Amazon suggests certain products to you, or why you only see certain information on your Facebook. We think there are ways to bring this research to the personal level by designing experiments individuals can run to speculate about how these algorithms work. Building on Henry Jenkin’s idea of “Civic Imagination”, we could ask people to design how they would want the algorithms to work, and perhaps develop descriptive visual explanations of their own ideas.
Get Involved!
We think each of these three can help build big data literacy and try to address big data’s empowerment problem. Read the paper for some other ideas. Do you have other ideas or experiences we can learn from? We’ll be working on some of these and look forward to collaborating!
You must be logged in to post a comment.